Design and implement machine learning algorithms to predict cancer patient drug response and identify “driver” ncRNA genes. –Supported by NIH/NCI 1R01CA282704 (Yang, D, impact score 24, percentile: 13%).
It is conceivable that if some ncRNA genes are repeatedly targeted by somatic chromosome copy number alterations or DNA methylation in cancer samples, these ncRNAs may play an important role in cancer initiation and progression. My group have developed computational algorithms to understand how ncRNA expression is somatically interrupted in the cancer genome and epigenome during tumor initiation and progression. I have led the ncRNA analysis in TCGA colorectal cancer, gastric cancer, low-grade glioma and melanoma working groups to characterize the ncRNA regulatory network and subtype by using these methods (Nature, 2012; Cell, 2015; N Engl J Med. 2015).
In 2018, we integrated multiple dimensional pharmacogenomic data of 11,950 lncRNAs in 5,605 tumors and 505 cancer cell lines to build machine learning algorithm to predict cancer patient drug response and identify “driver” genes (Fig. 5). Using Elastic Net (EN) regression model, we have successfully built lncRNA-drug response models for 265 anti-cancer agents across 27 cancer types. Our analysis identified 27,341 pan-cancer lncRNA-drug predictive pairs. We have shown that cancer cell line based lncRNA EN-models can predict therapeutic outcome in cancer patients. Further lncRNA-pathway co-expression analysis identified ADME chemo-resistance lncRNAs could regulate drug response through drug-metabolism and disposition pathway (Nat Commun, 2018).
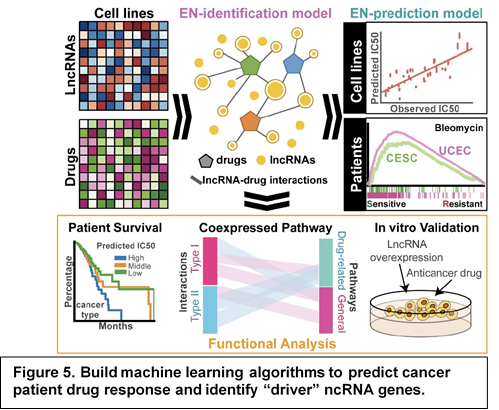
- Wang Y, Wang Z, Xu J, Li J, Li S, Zhang M, Yang D#. Systematic Identification of Non-coding Pharmacogenomic Landscape in Cancer, Nat Commun, 2018 Aug 9;9(1):3192. doi: 10.1038/s41467-018-05495-9.
- TCGA Network(including Yang D). Comprehensive molecular characterization of human colon and rectal cancer. Nature. 2012 Jul 18;487(7407):330-7. PubMed PMID: 22810696; PubMed Central PMCID: PMC3401966.
- TCGA Network(including Yang D). Genomic Classification of Cutaneous Melanoma. Cell. 2015 Jun 18;161(7):1681-96. PubMed PMID: 26091043; PubMed Central PMCID: PMC4580370.
- TCGA Network(including Yang D). Comprehensive, Integrative Genomic Analysis of Diffuse Lower-Grade Gliomas. N Engl J Med. 2015 Jun 25;372(26):2481-98. PubMed PMID: 26061751; PubMed Central PMCID: PMC4530011.